DevUseOps: architecting enterprise IT for autonomous business processes
- Prashanth Pappu
- Aug 6, 2024
- 4 min read
Updated: Dec 2, 2024
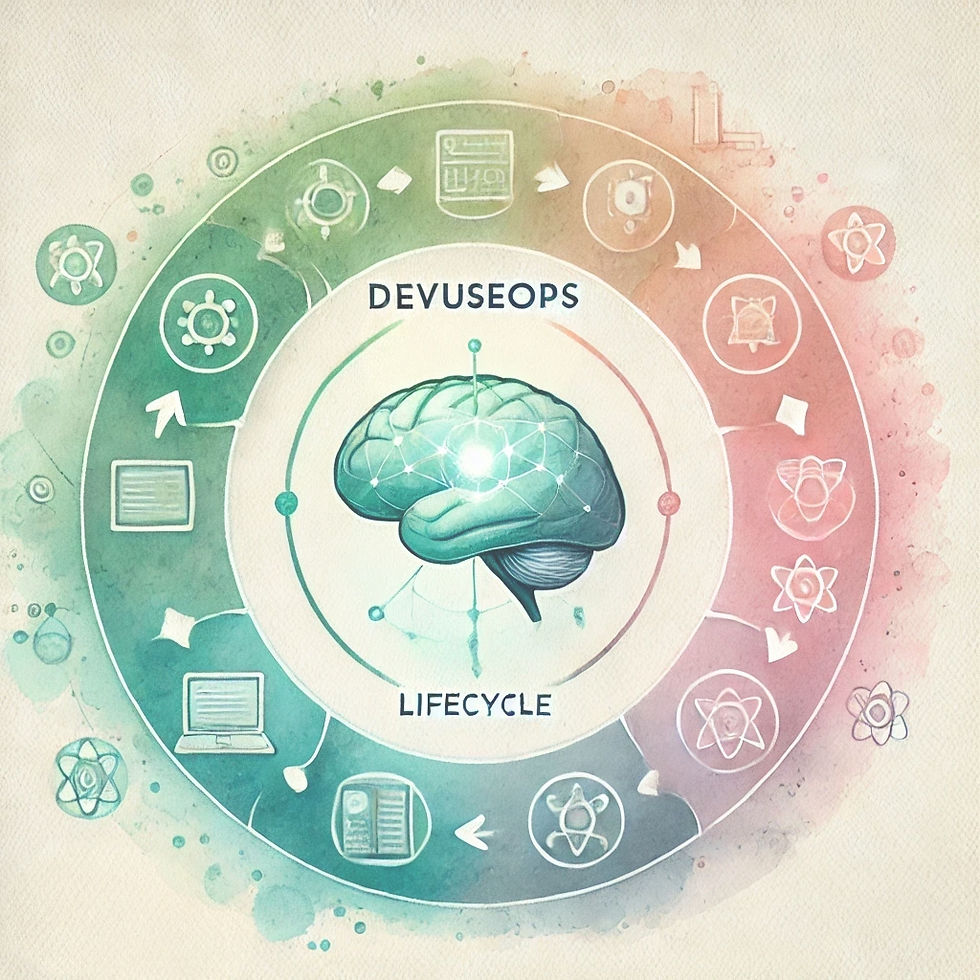
Tell me if you've heard this one. You know GenAI is foundational. You have a capable AI team and also a reputed IT services vendor. You get them together to execute a small AI project. The demo is fantastic! The CEO and the board loved it. The magic is palpable and even with feet firmly on the ground, they can see AI paving the way to improved business margins.
Demo Ready vs Enterprise Ready
It's time to productionize the demo and drive real enterprise usage and benefits. At the beginning, the issues are minor. AI fails on some scanned documents and your IT vendor says the fix is on the roadmap. Then an enthusiastic enterprise early adopter shoots off an email to multiple executive leaders with a list of complaints. AI added two zeros to net contract value and but for him it wouldn't have been caught! AI suggested list of suppliers is too pedestrian and not really being used by the procurement team. AI is missing key checks for the compliance team and they have to redo the work for a critical business milestone! Suddenly the complaints grow into a chorus: the teams do not know whether AI has done a good job or not and have to manually execute all the processes anyway.
Your teams begin to dread the word GenAI. The enthusiasm for AI demos is replaced with skepticism about real enterprise ROI.
Composition is Easy. Tuning is Hard.
Too many CTOs and CIOs have been through the above experience. The early years of GenAI have chiefly been times of show-piece demos that aren't quite enterprise ready.
The core problem, ironically, is that AI applications and workflows are easy to develop or compose. There are vendors, AI python engineers and IT services team that can easily compose complicated AI pipelines with low-code scripts. In fact, AI itself makes it easy to compose AI pipelines!
But alas, composition is easy yet tuning is hard.
AI pipelines and workflows composed with 10s of steps have a problem of compounding unreliability. Errors at any stage result in exponentially increasing errors in subsequent steps. So each of the 10 steps needs to be tuned for a high degree of accuracy before the workflow is even barely usable in enterprise environments by rank and file employees. The enterprise specific customizations that differentiate and make your business unique are exactly the things that trip up AI and call for even greater attention in tuning.
DevOps to DevUseOps
The challenge is equal parts technical and organizational. Unlike traditional IT deliverables like web sites and mobile apps, autonomous business processes require a deeper change in the enterprise organization and culture. Enterprise IT has largely been structured around the idea of DevOps. I.e., internal or outsourced vendor develops the custom enterprise application and another team supports and addresses any occasional operational issues with the applications.
Autonomous business processes require a significant organizational change from DevOps to DevUseOps.
DEV for intuitive AI collaboration : First, the internal or external development teams need to design autonomous business processes with a goal to foster AI+user collaboration and not user displacement by AI. Put simply, too many AI demo projects focus on ML programmers who build backends and create frontends as an afterthought. This not only results in a poor user experience but is a profoundly wrong approach. Autonomous business processes need newer UX paradigms that enables seamless AI+user collaboration while being compatible with your existing business processes where the user can get productivity benefits even if the AI does only 80% of the job or makes occasional errors.
USE for seamless AI feedback : Second, no matter how good your internal AI team or your vendor's AI platform is, it is impossible to capture the nuances of (a) your custom data (b) your custom business requirements and (c) your enterprise team's experience and cognitive judgment in your varied business processes. So the best training examples and feedback for AI are actually obtained when your real employees, and not some expert developers or designated specialists, actively check, correct and consult with the AI in an organization where user collaboration with autonomous business processes is the new normal.
OPS for continuous AI learning : Third, the entire success of your autonomous business processes depends on how quickly AI learns from the user feedback with continuous tuning. Manual log analysis, more ML expert time change requests, quarterly model updates are signals that the organization and technology platform are not set up for robust tuning required for every single node of your complex autonomous business processes. Automated conversion of implicit feedback from user+AI collaboration into rich and readily usable artefacts for continuous AI training is a foundational requirement to drive real enterprise value from autonomous business processes.
CTO's Checklist
The transformation of digital enterprises into autonomous enterprises is not just a possibility but an imperative for business sustainability and success in the AI age. The transformation requires newer technology platforms and also newer technology organizations. If you are a CTO or CIO actively addressing the challenge of realizing the benefits of autonomous business processes, here's a two question checklist:
Are you using a technology platform for your autonomous business processes that excels at every stage of the DevUseOps cycle?
Are you actively re-architecting your internal teams and outsourced vendors to build competencies and an organizational culture for DevUseOps?
Talk to us to learn how AiUi Flexflow can help address these challenges and realize the promise of Autonomous Enterprises.